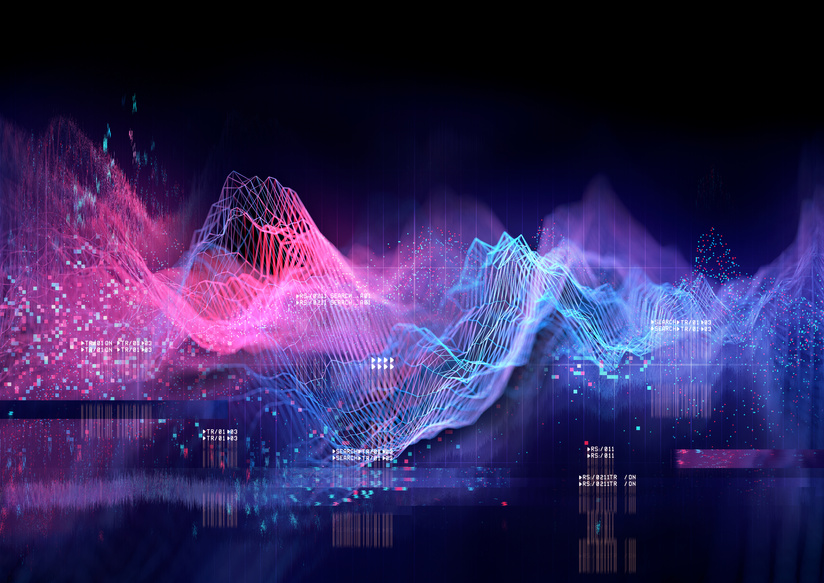
Posted by
Mordy Oberstein
There’s this sentiment that rank has become increasingly more volatile. To a large extent, we’ve chalked this up to machine learning, or RankBrain in specific. That said, what does the current Google ranking fluctuation landscape actually look like? How does it differ from the allegedly more stable past? What new ranking dynamics has machine learning left us with? What can we do about them?
This is going to be a two-parter. So, here’s the plan. In this post, we’ll lay out the rank landscape and start to delve into what it all means. In the piece that follows, we’ll pick apart the dynamics of ‘rank’ as it currently exists and how these dynamics influence how we approach determining what does and what does not work for ranking on the Google SERP.
For now, though, join me as we go down the rabbit hole that is rank stability.
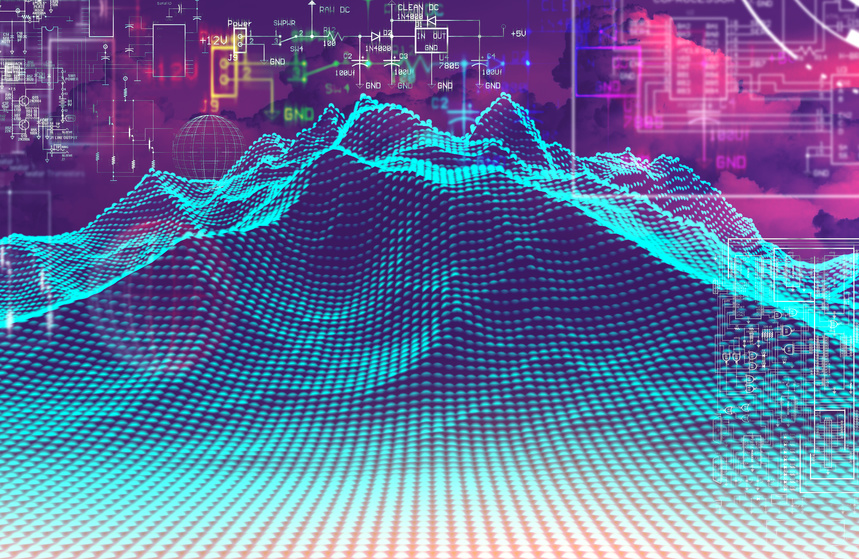
How Much Do Google Rankings Fluctuate?
That’s a great question, and the answer is …. it depends. It depends on “when” we’re talking about. There is no magic number that we can assign to rank fluctuations, it’s relative. That is, the question is not how much does rank fluctuate, but rather, how much more (or less) does rank fluctuate now as compared to the past.
Indeed, this is how Google rank fluctuation weather tools, such as our own Rank Risk Index, operate. Whether high or low fluctuations are recorded depends on previous rank behavior. Should rank fluctuate excessively more today than yesterday, you’ll see a spike on the index indicating high levels of rank fluctuations. But what if rank fluctuations are always high? Then what? Well, then the index will record normal levels of shifts in the rankings. The point being, tools that track rank fluctuations cannot tell you if rank is consistently volatile and ever-changing. All they can tell you is if rank is more volatile than it had been over the past 20 – 30 days (which is why they’re designed to track aberrations, i.e., Google updates, and not routine rank behavior, no matter how volatile that behavior may be).
So, let’s rephrase this question to be: How much does rank fluctuate relative to the past?
How Much Rank Fluctuates: Then and Now
To answer this question we’re going to look at data across five niches (i.e., Travel, Food and Drink, Business Services, Real Estate, and Technology and Computing), that when aggregated come out to approximately 5,500 keywords. Specifically, I used a metric called Top 5 Results – Exact Match. What this means is I looked at the number of queries that produced the same exact top 5 results in the same exact order from one month to the next.
Allow me to elaborate. Say I ran 100 queries on January 1st and tracked which domains appeared in the top five results shown on the Google SERP for each keyword. Say I also tracked the order that they appeared in. Comes February 1st, and I decided to run the very same queries again and to track them the same way. Now, with two data sets, I go about comparing which queries on February 1st had the same domains in the same exact order in the top 5 when compared to the very same searches done on January 1st. For argument’s sake, let’s just suppose 50 of the queries I ran on February 1st showed the same domains, in the same order, as they did on January 1st. In such a scenario we could say that 50% of the queries ran in February had the exact same top 5 results and in the same exact order… or… Top 5 Results – Exact Match.
This is exactly what I did, except that it was pretty much all automated, i.e., no one was sitting with a magnifying glass comparing thousands of domains/results to each other. In specific, I collected data from three different points, April 2016, April 2017, and April 2018 and recorded the percentage of keywords where the top 5 results were an exact match (to what was recorded in the previous months, i.e., March 2016, March 2017, and March 2018).
Here’s what I came back with:
In a nutshell, 2016 was far more “stable”
Year-to-year, the numbers are staggering:
Between 2016-2017 rank stability within the top 5 results dropped by 45%! The following year was a bit more stable (at least as far as this metric is concerned). From 2017-2018 there was a 32% drop in the number of exact matches within the top 5 results. Overall, from 2016-2018 there was a 39% drop in rank stability within the top 5 results on the Google SERP, with the Business Services niche taking the largest hit by posting a 51% top 5 exact match loss.
It should be noted that I did an initial dive into the top 1 and top 3 results as well, looking at the exact matches produced by those ranking positions. As I’ve reported in the past, the closer to the top of the SERP, rank, as is to be expected, is more stable. That is, there are fewer fluctuations in the top ranking position than are found at the fourth spot on the SERP. Thus, the above data does not mean that each position within the top 5 fluctuated to an equal extent.
In either event, we’re just getting started here. While we have determined that rank is fluctuating far more often than it had in the past (and if you think the top 5 are bad, let’s not even talk about the top 10 – which I’ve also reported on in the past), we don’t know to what extent sites are fluctuating on the SERP.
Check out our guide to dealing with SERP fluctuations.
How Many Positions Do Results Fluctuate?
With the awareness that rank fluctuations are far more common today than in the past, it behooves us to analyze the extent of these rank changes. How significant is the increase in rank fluctuations? The increase in rank fluctuations between 2016 and 2018 has to be qualified. In simple terms, how many positions does a site tend to move when it is in fact on the move?
Average Position Change Data
To help qualify the increase in rank fluctuations previously noted, I pulled data showing the average number of positions results within the niches shifted between 2016 and 2018. In this instance, the data only reflects the top 20 results for any given keyword within any of the niches. As such, the data I am about to present reflects the average number of positions that results typically found on the first two pages of the SERP tended to shift. Here, both the specific number of position changes and the overall trend are important to note.
Without further adieu, here is the data:
In 2016, domains within the top 20 organic results (for the keywords within the dataset) tended to shift 2.46 positions on average (when in fact they did shift
What’s important though is the trend. Is rank more or less stable? It’s hard to make the case for increased stability when in both 2017 and 2018 the average position change across all niches jumped. By April 2018 we had moved from an average swing of two positions to movement just above 4 results. That’s a 70% increase when comparing 2018’s data to that of 2016.
When looking at the year-to-year data, another trend emerges:
Like the data for the Top 5 Results – Exact Match metric, here too the change between 2016-2017 was considerably greater than the year-to-year changes seen from 2017-2018. The Travel niche saw its average position change increase by 55% from 2016-2017, whereas between 2017-2018 there was “only” a 13% increase. This was true for all niches except Food and Drink, which saw a slight increase in its position movement from 2017-2018 as compared to the previous period.
What’s really interesting to me is that the Real Estate niche did not see as vast an increase in average position change from year-to-year when compared to the other industries. This is all the more fascinating when you look at where the niche started. The 2016 data was already high, the highest of all the niches, with an average position change of 2.99. So, you have a niche that relative to other industries already displays a high average position change in 2016. What follows is an increase in that average, but one that is not as steep as its counterparts. The result is an average position change in April of 2018 that falls right into the position movement range of the other niches. Coincidence or not, it is interesting.
AI is Driving Ranking Trends and Fluctuations
There’s a pink elephant in the room, an 800-pound gorilla named RankBrain. Google’s AI superstar came on the scene in 2015 and since then it’s grown… because that’s what machine learning is supposed to do. The entire purpose of introducing RankBrain was for it to learn more and extend its impact on search results. Meaning, at the onset, RankBrain had limited reach, however, it’s now thought that it touches nearly every query, and almost as often influences rank itself.
The timing works out well then. That is, just as RankBrain started to get its feet wet back in 2016, rank took on a much more volatile trajectory. It’s not an accident that rank becomes far less stable with the advent and proliferation of RankBrain. In other words, as Google’s machine learning paradigm started to get a grasp on user intent, the rankings shifted accordingly. And they continue to shift.
The question is not why is rank more volatile than it once was. The real question is how is it that rank is so consistently volatile? An adjustment to the algorithm cannot explain SERP volatility as the norm. The amount of “hand-crafted” adjustments would be unfathomable. The only way to maintain high levels of rank fluctuations on a consistent basis is via RankBrain and Google’s other machine learning properties.
That’s why the data between April 2016 and that of April 2017 breaks the way it does. This was a breakthrough period for RankBrain where it took off and impacted rankings. That’s why volatility has only increased from 2017-2018. In fact, rank volatility has increased between 2017-2018, but if you look back at the data above, not at the same breakneck speed. It would appear that RankBrain had an enormous initial impact that has time went on slowed just a tad as the gap that RankBrain was meant to bridge has narrowed with its very expansion. In other words, when RankBrain came on the scene in earnest (circa 2016), the Google SERP was so “unrefined” (from an intent parsing perspective) that the initial corrections were “abnormally” visible and large (i.e., RankBrain had a lot of work to do). As time went on and goes on, we have not and I suspect we will not see rank volatility at those levels again. However, as RankBrain’s very nature is continual and evolving, fluctuations should continue to increase as it improves on its own methods. Which is exactly what has happened.
Simply, the data and trends shown above align with RankBrain and its trajectory.
An Enormous Increase in Rank Diversity
I’ve been keeping a wild card up my sleeve this whole time. Rank diversity. One of the most pronounced changes I’ve noticed since the era of RankBrain came upon us relates to rank diversity. That is, with the understanding of user intent, with Google being better able to parse user intent, with Google recognizing more diversity within queries inherently comes more diversity. A more diverse understanding of intent, a more diverse set of sites to satisfy such a diverse spectrum of “user wants.”
Quite logically, a more nuanced and layered understanding of user intent results in a more diverse SERP that contains more diverse organic links. Simply, the more diverse Google’s understanding of keyword intent is the more diverse set of domains the search engine is going to work towards. Considering that very point, the below data on unique domains showing on the SERP should not surprise you:
The data above reflects how many unique domains Google showed within all of the results for all of the keywords tracked within a given niche’s dataset. To be clear, multiple pages from the same domain would count as one unique domain within this metric. What you see here then is an outstanding shift towards rank diversity in 2017 and beyond.
Of all the metrics shown in this study, the shifts seen within the Total Unique Domains are the most dramatic. Overall, between 2016 and 2018 there was a nearly 70% increase in the number of new unique domains across all of the niches studied.
The year-to-year data set is even more interesting:
You can see a huge percentage increase between 2016-2017 as a slew of new domains entered the ranking mix (which of course makes
What Does Machine Learning’s Impact on Rank Actually Look Like?
The novelty in the above is not in the assertion that machine learning has made rank more volatile. Rather, the data above helps to paint a picture as to the extent to which properties such as RankBrain have impacted the
I am going to be “brief” about this here. I’ll lay out the general outline of how I see machine learning changing the ranking ecosystem, but get into the nitty-gritty of it all in the follow-up post, or “Part II.” For now, though, let’s skim the surface of “intent” and how Google’s ability to better parse it has changed the “ranking terrain” that is the SERP.
Machine Learning’s Multifaceted Understanding of Intent & How It Impacts Rank
There
Take the following set of results for the keyword
As you may have noticed, there are two types of sites on this page, those that offer car insurance policies (highlighted in blue) and those that offer information about buying car insurance (boxed in red). In fact, 4/10 of the organic results here are these informative sites, or 40% of the SERP for the keyword buy car insurance are sites where you cannot, in fact, buy car insurance.
That is, Google, via its machine learning properties (in this case I would assume primarily RankBrain), has determined that the user has two possible intents when looking to buy car insurance; to actually buy a policy and/or to learn about doing so.
So what?
Ranking Before Optimization
The above is actually a very big deal. It means, that prior to getting to a site’s optimization per se, its ability to rank or not to rank is heavily determined by Google’s machine learning properties.
How so?
Well, in our case here, sites that sell car insurance only have access to 60% of page one of the SERP. That is, no matter how optimized a particular site may be, there is no way it can access more than 6 of the 10 organic results on page one of this SERP if the said site sells car insurance. In other words, it’s not that machine learning has made rank more volatile and now you need to better ensure that you offer Google a quality site to crawl, and so forth. Rather, machine learning has made a transformative change in that it has altered the path sites must undergo before arriving on the SERP.
In an environment where rank is determined, to a large extent, by how Google understands the latent intent of a keyword in relation to the intents(s) embedded within site content, there is a tremendous opportunity for volatility. That is, where volatility depends on Google manually altering the weighing of any given number of ranking factors, or adjusting its traditional algorithms, volatility is to a large extent the exception, not the rule (I mean that from a systemic point of view).
Such is not the case when intent, and ever-improving ability to understand is the driving force behind rank. As Google recalibrates how it understands
Think of it like this, say Google decides that when you search for buy car insurance that the user means to solely learn about buying a car insurance policy, not to actually buy one. What then happens to those six sites sitting on page one that
In real terms, this would mean that two sites that offer car insurance would find they no longer rank on page one, while two informative sites would see their rankings shoot up the SERP, all thanks to machine learning.
As Google’s machine learning does what it is supposed to do, i.e., learn, sites will inherently move more often than they used to. But is there anything to do?
How to Best Navigate A More Volatile Rank Environment
The short answer is experimentation. If Google is always trying to improve how it understands